The Vera Rubin Observatory Legacy Survey of Space and Time (LSST) is expected to detected 10 million alerts per night for a total run of 10 years.
Fink is one of the community brokers chosen to receive this full alert stream, filter, add value and redistribute a variety of sub-streams to the astronomical community.
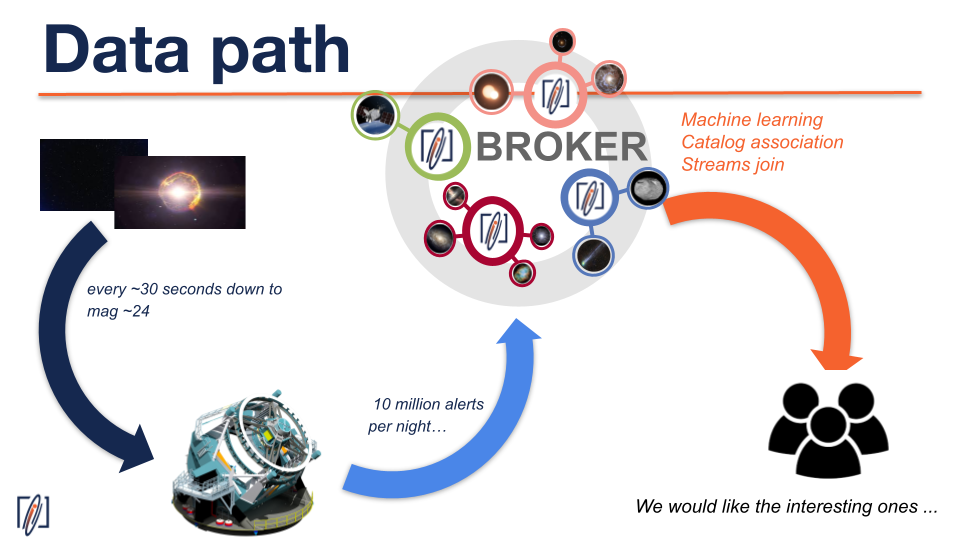